Hello all, and welcome back to MedTech Compliance Chronicles. We have been on our journey of bringing a medical device to market in the United States for quite some time now. A significant portion of this journey has been investigating the quality (management) system requirements. Good news! We are nearing the end of these requirements!
One of the final requirements surrounding the QMS is regarding statistical techniques and analysis of data. This is a very broad topic and one that will not be covered in a single post, indeed, there are PhD’s given on any one topic that might fall under ‘statistical techniques.’ In today’s post, I will present the general requirements around statistical techniques and analysis of data and present some commonly used techniques in the medical device industry. I will not go into detail on any particular technique. However, I understand that this may be helpful to my readers and so I will, from this point on, include a post on a particular statistical technique in detail every 2-4 posts. Without further ado, let's jump right into it!
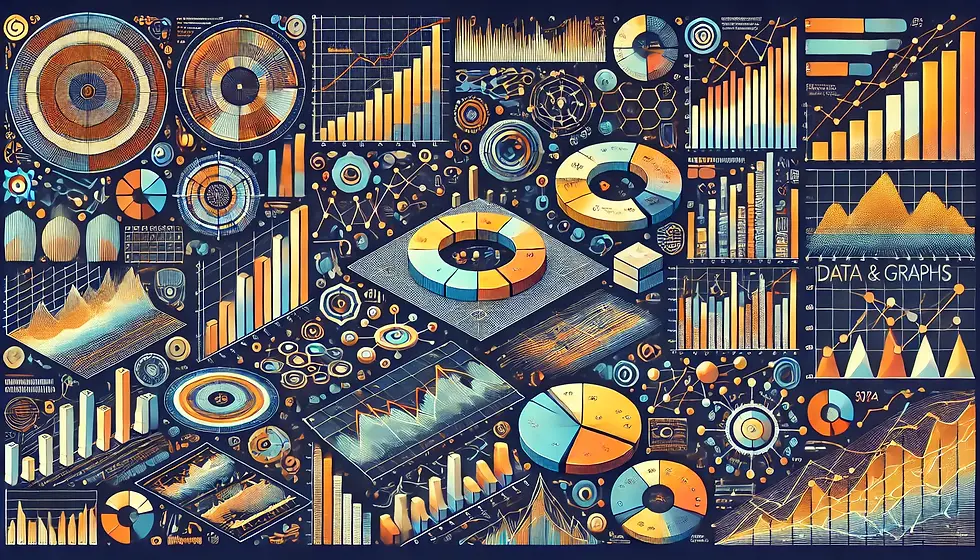
Requirements
The actual requirements regarding statistical techniques from the US FDA are quite sparse considering the importance proper selection of statistical technique has on the validity of pretty much any study performed. However, the sparseness of the requirements is also necessary given the fact that statistical techniques are almost as numerous as types of medical devices and so one-size-fits-all requirements simply are not effective. The requirements essentially boil down to you must select “valid” statistical techniques to ensure the capability of your processes and verify your product characteristics. The second part of the requirement is essentially the same, just specific to sampling plans. You must select “valid” sampling plans for all sampling activities.
The key word in both requirements is “valid.” Valid means that there is documented rationale for why the specific technique or sampling plan was chosen and that rationale is based on generally accepted statistical principles. There are a couple of things that you must know in order to determine the validity of a technique or sampling plan. The first and foremost, as with most things quality, is risk. In statistics, there are numerous options for basically everything you would want to test and differing degrees of sample sizes required for various ‘powers’ (a statistical term for the probability of rejecting a false null hypothesis, often used to determine required sample sizes) of the test. The different tests will often be determined by the level of sensitivity or robustness required and the type of data being analyzed. You must draw a line and choose a test and sample size somewhere and the decision should be based on risk. What is the risk to the finished device if this statistical test fails to detect what it is meant to? The organization should have a procedure which documents the statistical methods and sampling plans used in relation to risk. For example, the procedure could state that when statistical techniques are applied to low risk issues a confidence level of 90% is required with a power of 80%. While medium risk issues may require a confidence level of 95% and/or a power of 90%.
Beyond risk, what determines whether or not a statistical technique is valid is primarily based on its appropriateness for the particular application. For an ultra simplified example, you cannot use a binomial distribution to model continuous data. Therefore, if your procedure mentions that the binomial distribution is used but the data type being analyzed is continuous, this would not be considered a valid technique. Unfortunately, it is not really possible to get much more specific than this in a blog post as the statistical validity of a particular technique is heavily dependent on what it is being applied to. However, the FDA does have several guidance documents on the application of statistical techniques to specific scenarios so if you are having a rough time choosing one give the FDA guidance documents a search.
That is basically it for US FDA requirements. ISO 13485:2016 goes a little deeper by requiring that data from specific sources be continually analyzed. These sources are; (customer) feedback, conformity to product requirements (i.e. inspection results, nonconformities, CAPAs, etc.), characteristics and trends of processes and product, suppliers, audits and service reports as applicable. All medical device manufacturers are already required to collect this data via supplier controls and incoming and in-process inspections, monitoring of critical process parameters, FDA and ISO audits, etc. This requirement is just to ensure that manufacturers are actually doing something with the data, not just collecting it and never looking at it again.
Common Statistical Techniques
There are numerous statistical techniques that each can be incredibly useful given a specific situation, far too many to cover in a single blog post. Here I will give a brief overview of the most fundamental statistical techniques that pretty much all organizations will actually need to apply at some point. Those techniques are; sampling, design of experiment and hypothesis testing.
Sampling is what we call it when we take a small piece of an overall population to test and then make assumptions about the whole population based on the results of the sample. Unless you intend on inspecting 100% of everything that comes in and goes out of every process, you will need to use some sort of sampling. Thankfully, and sometimes unfortunately, sampling in manufacturing industries has several of its own international standards. These standards provide tables that, if properly interpreted, will tell you the exact sample size necessary for statistical validity based on the situation. I said that this is sometimes unfortunate because the statistical validity of the sample size is dependent upon proper interpretation of the tables which requires some degree of fundamental understanding of the principles behind sampling and how the tables were constructed. Many users of the standard tables, however, simply pick a random table that displays their lot size and take a sample based on what it says. The standards do a pretty good job of explaining the principles used to create the tables and how to properly interpret them so if you actually read them and do not just skip to the tables, you should be in good hands. Another important consideration when sampling is to consider the randomness of your samples and how that might affect the results you are trying to get. I will leave details on types of sampling and randomization for a post specifically on sampling.
The next form of statistical technique used quite frequently in medical device manufacturing is factorial experiments, which in industry is often used under the more scientifically leaning term, design of experiment. Design of experiment (DOE) is used to systematically plan experiments to investigate the effects of multiple factors on a process or product. It helps in identifying the relationship between factors affecting a process and the output of that process. In practice, it is used in process development to find critical process parameters to monitor and set the process limits. DOE is also used when qualifying new equipment to determine optimal operating ranges of the equipment. It is also a critical tool in any process improvement effort, root cause analysis and even product development.
The final statistical technique with pretty universal applicability is hypothesis testing. Hypothesis testing, sampling and DOE go hand-in-hand in many cases. You design an experiment, take samples to test for that experiment and then perform a hypothesis test on the results of that experiment. When performing a hypothesis test, you will have some quantity about your product or process which you wish to test against some other known quantity. The hypothesis will generally be in the form of the tested quantity is likely equal to, not equal to, less than or greater than the known quantity. The test quantity could be a range of things such as the dimensions of a specific measurement, peel strength of a sterile barrier system, proportion of correctly identified defects in a visual inspection, etc. The known quantity could then be things such as the specification limits, historical performance levels, expected performance levels (in the case a hypothesis test is testing the results of an improvement effort or a new process), an international standard, etc. Basically, anytime you perform an experiment, you will have some type of expected results. Hypothesis testing is how you verify how well the actual results match up to your expected results.
Conclusion
Overall, statistical techniques and analysis of data are an integral part of a quality management system. If you have been following along in our QMS blog series, you will notice a trend of requirements for objective evidence for pretty much everything (design and development outputs, process development and control, inspections, etc.). Statistical techniques and analysis of data are the primary tools which medical device manufacturers use to provide the objective evidence required in most cases. So while the requirements themselves may seem short and sweet, they are actually quite intricate and something that must be thought of every step of the way. Think about it like this, any time objective evidence is a requirement, you will either need to produce said evidence for every single product, process run or whatever the area of interest is OR, you will need a valid statistical rationale for selecting a subset of the group (a sample) to provide the objective evidence for.
Bình luận